9. Stochastic Methods: Exercise#
Name:
import numpy as np
import matplotlib.pyplot as plt
%matplotlib inline
1. Integration#
When an explicit function like \(x_n = g(x_1,...,x_{n-1})\) is not available, you can use a binary function that takes 1 within and 0 outside of a region to measure its volume.
Measure the volume of an n-dimensional sphere using a binary function
Measure the area or volume of a shape of your interest by sampling method.
2. Rejection Sampling#
Let us generate samples from Gamma distribution
with the shape parameter \(k>0\) and the scaling parameter \(\theta\) using the gamma function \(\Gamma(k)\) available in scipy.special
.
from scipy.special import gamma
from scipy.special import factorial
Here is how the gamma function looks like, together with factorial at integer values.
kmax = 5
x = np.linspace(0, kmax, 50)
plt.plot(x, gamma(x))
plt.plot(range(1,kmax+1), [factorial(k-1) for k in range(1,kmax+1)], 'o')
plt.xlabel("x"); plt.ylabel("f(x)")
Text(0, 0.5, 'f(x)')
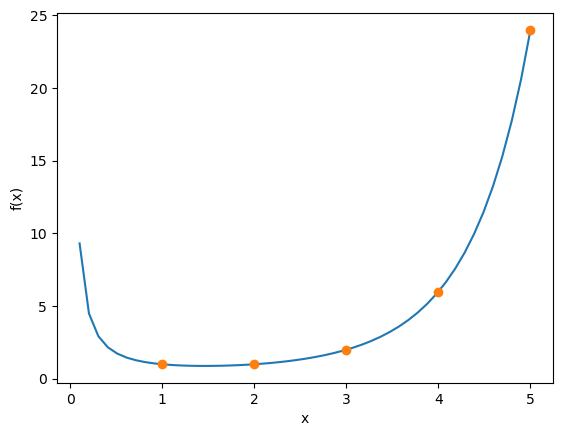
Define the Gamma density function with arbitrary \(k\) and \(\theta\).
def p_gamma(x, k=1, theta=1):
"""density function of gamma distribution"""
return
Consider the exponential distribution \(q(x;\mu)=\frac{1}{\mu}e^{-\frac{x}{\mu}}\) as the proposal distribution.
def p_exp(x, mu=1):
"""density function of exponential distribution"""
return
def x_exp(n=1, mu=1):
"""sample from exponential distribution"""
y = np.random.random(n) # uniform in [0,1]
return
The ratio of the target and proposal distributions is
By setting
we have
Thus at
the ratio \(\frac{p(x;k,\theta)}{q(x;\mu)}\) takes the maximum
What is a good choice of \(\mu\) to satisfy \(p(x)\le cq(x)\) and what is the value of \(c\) for that?
By setting \(\mu=k\theta\), we have \(p(x)\le cq(x)\) with \(c=\frac{k^k}{\Gamma(k)}e^{1-k}\)
Verify that \(cq(x)\) covers \(p(x)\) by plotting them for some \(k\) and \(\theta\).
k = 2
theta = 2
c = (k**k)/gamma(k)*np.exp(1-k)
x = np.linspace(0, 10, 50)
plt.plot(x, p_gamma(x, k, theta))
plt.plot(x, c*p_exp(x, k*theta))
plt.xlabel("x");
---------------------------------------------------------------------------
ValueError Traceback (most recent call last)
Cell In[6], line 5
3 c = (k**k)/gamma(k)*np.exp(1-k)
4 x = np.linspace(0, 10, 50)
----> 5 plt.plot(x, p_gamma(x, k, theta))
6 plt.plot(x, c*p_exp(x, k*theta))
7 plt.xlabel("x");
File ~/miniforge3/lib/python3.13/site-packages/matplotlib/pyplot.py:3827, in plot(scalex, scaley, data, *args, **kwargs)
3819 @_copy_docstring_and_deprecators(Axes.plot)
3820 def plot(
3821 *args: float | ArrayLike | str,
(...) 3825 **kwargs,
3826 ) -> list[Line2D]:
-> 3827 return gca().plot(
3828 *args,
3829 scalex=scalex,
3830 scaley=scaley,
3831 **({"data": data} if data is not None else {}),
3832 **kwargs,
3833 )
File ~/miniforge3/lib/python3.13/site-packages/matplotlib/axes/_axes.py:1777, in Axes.plot(self, scalex, scaley, data, *args, **kwargs)
1534 """
1535 Plot y versus x as lines and/or markers.
1536
(...) 1774 (``'green'``) or hex strings (``'#008000'``).
1775 """
1776 kwargs = cbook.normalize_kwargs(kwargs, mlines.Line2D)
-> 1777 lines = [*self._get_lines(self, *args, data=data, **kwargs)]
1778 for line in lines:
1779 self.add_line(line)
File ~/miniforge3/lib/python3.13/site-packages/matplotlib/axes/_base.py:297, in _process_plot_var_args.__call__(self, axes, data, return_kwargs, *args, **kwargs)
295 this += args[0],
296 args = args[1:]
--> 297 yield from self._plot_args(
298 axes, this, kwargs, ambiguous_fmt_datakey=ambiguous_fmt_datakey,
299 return_kwargs=return_kwargs
300 )
File ~/miniforge3/lib/python3.13/site-packages/matplotlib/axes/_base.py:455, in _process_plot_var_args._plot_args(self, axes, tup, kwargs, return_kwargs, ambiguous_fmt_datakey)
452 # Don't allow any None value; these would be up-converted to one
453 # element array of None which causes problems downstream.
454 if any(v is None for v in tup):
--> 455 raise ValueError("x, y, and format string must not be None")
457 kw = {}
458 for prop_name, val in zip(('linestyle', 'marker', 'color'),
459 (linestyle, marker, color)):
ValueError: x, y, and format string must not be None
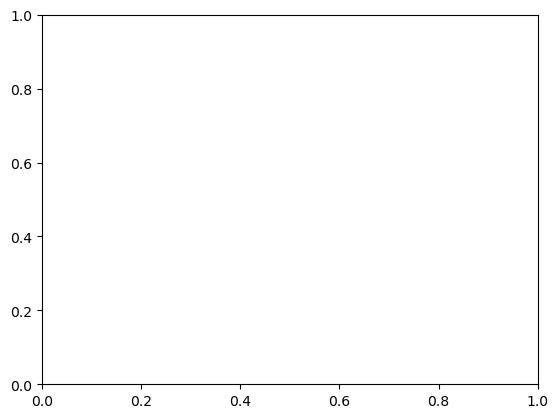
Implement a function to general samples from Gamma distribution with arbitorary \(k\) and \(\theta\) using rejection sampling from exponential distribution.
def x_gamma(n=1, k=1, theta=1):
"""sample from gamma distribution by rejection sampling"""
c = (k**k)/gamma(k)*np.exp(1-k)
#print("c =", c)
xe = x_exp(n, k*theta)
paccept =
accept = np.random.random(n)<paccept
xg = xe[accept] # rejection sampling
#print("accept rate =", len(xg)/n)
return(xg)
k = 2
theta = 2
# sample histogram
xs = x_gamma(1000, k, theta)
plt.hist(xs, bins=20, density=True)
# compare with the density function
x = np.linspace(0, 10, 100)
plt.plot(x, p_gamma(x, k, theta))
plt.xlabel("")
3. Importance Sampling#
You have \(m\) sample values \(f(x_i)\) \((i=1,...m)\) at \(x_i\) following a normal distribution
Consider estimating the mean of \(f(x)\) for samples with a different normal distribution \(p(x;\mu_1, \sigma_1)\) by importance sampling
What is the importance weight \(\frac{p(x)}{q(x)}\)?
Let us consider the simplest case of \(f(x)=x\).
Generate \(m=100\) samples with \(\mu_0=100\) and \(\sigma_0=20\) and take the sample mean \(E_q[f(x)]\).
m = 100
mu0 = 100
sig0 = 20
xs =
# show histogram
plt.hist(xs, bins=20, density=True)
plt.xlabel("x")
# check the sample mean
np.mean(xs)
Estimate the mean \(E_p[f(x)]\) for \(\mu_1=120\) and \(\sigma_1=10\) by importance sampling.
mu1 = 120
sig1 = 20
importance =
mean1 = np.dot(importance, xs)/m
print(mean1)
See how the result changes with different settings of \(\mu_1\), \(\sigma_1\) and sample size \(m\).
Optional) Try with a different function \(f(x)\).
4. MCMC#
Try applying Metropolis sampling to your own (unnormalized) distribution.